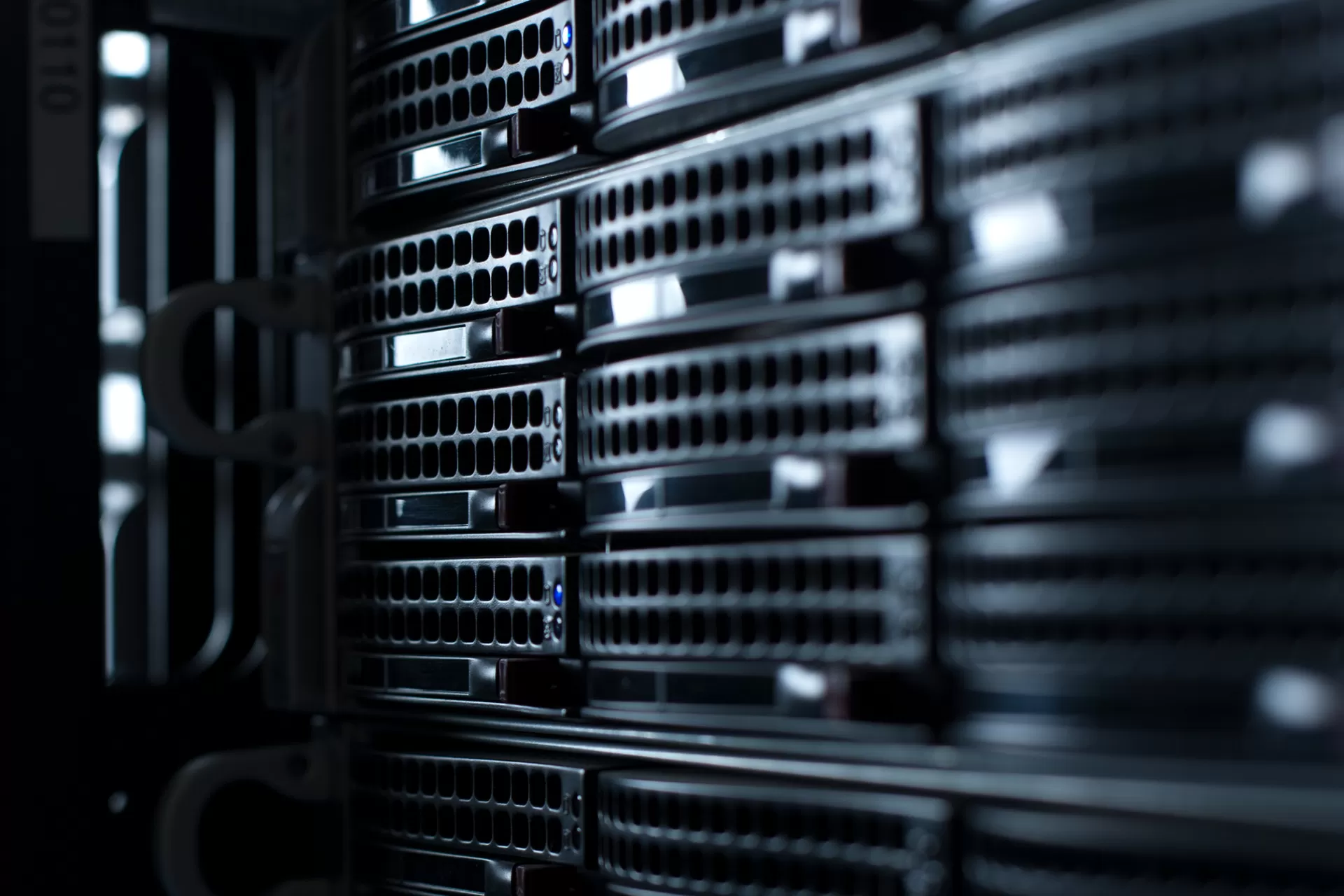
Intelligent and Digital Supply Chain and Manufacturing Processes using Oracle Adaptive Intelligence
Digital Automation is not new in today’s world. Most organizations use it or strive to use it. One area of Digital Automation is Artificial Intelligence, which can significantly enhance your ability to impact the business positively.
Gartner named Oracle a ‘Visionary’ in the 2020 Gartner Magic Quadrant for Analytics and Business Intelligence Platforms. Oracle’s platforms heavily use AI (Artifical Intelligence) and ML (Machine Learning) to provide accurate analysis and predictions. A similar model has been put to use in other areas, and one of the beneficiaries is the “Supply Chain and Manufacturing” module in Oracle ERP, known as the Oracle Adaptive Intelligence App.
Product Features
Below are some key features of the product and the phases required to configure it:
1. Data Acquisition and Storage
· Data from machines and equipment – Using IoT and sensors, data can be collected from the shop floor.
· Data from enterprise applications – Data can be collected from ERP, CRM, HCM, SCM with the help of interfaces.
· Embedded Data Management Platform – Data collected from various other sources, i.e., Data Lakes.
2. Data Contextualization and Preparation
· Operational Technology (OT) and IT data contextualization – Contextualize data and get a point-in-time view of the manufacturing process.
· Sensor-Time-Series Data – Aggregate using Symbolic Aggregate approXimation (SAX).
· 5M Data Preparation – Use attributes such as manpower, machine, method, material, and management for a comprehensive analysis.
3. Model Lifecycle Management
· Model creation – Build predictive models for analyzing critical KPIs.
· Model training and deployment – Constant process of training the models using historical data.
· Model performance evaluation – Evaluate the accuracy of the predictive model using the confusion matrix and compare predictions with actual results.
4. Patterns and Correlations Analysis
· 5M input factors – Analyze the data collected from manufacturing operations and understand the impact on major business outcomes.
· Top influencing factors – Identify factors having a high influence on KPIs.
· Patterns and correlations from historical data – Identify relationships between many influencing factors and the variables from manufacturing processes.
5. Predictive Analysis
· Critical outcomes during manufacturing – Compare current versus historical patterns.
· Prediction alert rules – Configure alerts to inform the user regarding a preset condition.
· Downstream orchestration – Use REST services to take action based on alerts, i.e., inform foreman if an abnormality is predicted.
6. Genealogy and Traceability Analysis
· Self-guided navigation for traceability – Intuitive and graph-based navigation can be used to trace the entire manufacturing process.
· Time-window traceability – View manufacturing events at any point in time, such as job on hold/released.
· Impacted products and customers – Trace forward impact of manufacturing factors on customers and products.
Use Cases
- Industrial automation is a buzz word, and the environment of the manufacturing process is changing significantly from the traditional manufacturing plant to a Smart Factory, that uses the IoT technology to its fullest. Sensors are at the core of any IoT solution. Each process on the shop floor is monitored by multiple sensors, which generate a massive amount of data. This data is transferred back to the data server or an application such as an Oracle ERP. This data has to be processed, digested, and stored. Such data stored is of no value unless there is a proper plan to analyze it and gain meaningful insights.
It can also be used for two purposes –
- Historical data, trends, and predictive analytics.
- Dynamic action to be taken in response to an event.
A typical scenario would be to identify and detect faults in the finished product. IoT can help identify the defects upfront, which would trigger an event. This event can use the previous data and can self-correct to ensure that the fault is fixed, thereby minimizing the production losses and deliver a good quality end product.
2. One major problem statement in the SCM area is shipping goods accurately and on time. Using AI, one can understand the shipping patterns, common issues faced, demand from a particular region, and optimize the entire logistics route to meet the needs. This can be done with minimal or no manual intervention, and the whole process will make the shipping faster and economical using the best possible routes. Apart from these business benefits, it will also help keep the customer happy by delivering the products on or before time.
Business Benefits
Below are some of the business benefits which can be reaped by using Oracle’s Adaptive Intelligence cloud app :
- Improve Production Yield
- Quality Product
- Increase Operational Efficiency
- Informed Decision Making
Oracle’s Adaptive Intelligence Cloud app is designed to achieve the above business benefits with the help of AI and ML. It is focused on providing the business with connected intelligence to achieve business outcomes.
Some of the value which this product brings are:
1. Gaining actionable insights into operational inefficiencies: Analyze key patterns and correlations that are related to manufacturing operations.
2. Performing rapid root-cause analysis: Trace manpower, machine, material, method, and management-related information.
3. Proactively address potential risks: Predict the probability of critical events and take proactive measures to address them.
4. Assess and mitigate business impact: Identify impacted products, processes, suppliers, and customers, and take actions to minimize risks.
Oracle Adaptive Intelligence can be used for other business areas such as Finance, Procurement, HCM, Marketing, and Sales.